Abstract
Background
The efficacy of epidermal growth factor receptor (EGFR)-tyrosine kinase inhibitor (TKI) was affected by numerous factors. In the study, we developed and validated an artificial neural network (ANN) system based on clinical characteristics and next-generation sequencing (NGS) to support clinical decisions.
Methods
A multicenter retrospective non-interventional study was conducted. 240 patients from three hospitals with advanced non-small cell lung cancer (NSCLC) and EGFR mutation were tested by NGS before the first treatment. All patients received formal EGFR-TKIs treatment. Five different models were individually trained to predict the efficacy of EGFR-TKIs based on one medical center with 188 patients. Two independent cohorts from other medical centers were collected for external validation.
Results
Compared with logistic regression, four machine learning methods showed better predicting abilities for EGFR-TKIs. The inclusion of NGS tests improved the predictive power of models. ANN performed best on the dataset with mutations TP53, RB1, PIK3CA, EGFR mutation sites, and tumor mutation burden (TMB). The prediction accuracy, recall and AUC were 0.82, 0.82, and 0.82, respectively in our final model. In the external validation set, ANN still showed good performance and differentiated patients with poor outcomes. Finally, a clinical decision support software based on ANN was developed and provided a visualization interface for clinicians.
Conclusion
This study provides an approach to assess the efficacy of NSCLC patients with first-line EGFR-TKI treatment. Software is developed to support clinical decisions.






Similar content being viewed by others
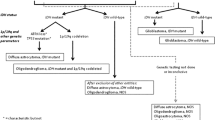
Availability of data and materials
Data are available upon request but may require data transfer agreements. Codes on shared on github (https://github.com/GuanRunwei/A-Clinical-Decision-Support-System-of-EGFR-TKIs).
References
Blons H et al (2021) PTEN, ATM, IDH1 mutations and MAPK pathway activation as modulators of PFS and OS in patients treated by first line EGFR TKI, an ancillary study of the French Cooperative Thoracic Intergroup (IFCT) Biomarkers France project. Lung Cancer 151:69–75
Canale M et al (2017) Impact of TP53 mutations on outcome in EGFR-mutated patients treated with first-line tyrosine kinase inhibitors. Clin Cancer Res 23(9):2195–2202
Chen YH et al (2019) Clinical factors associated with treatment outcomes in EGFR mutant non-small cell lung cancer patients with brain metastases: a case-control observational study. BMC Cancer 19(1):1006
Chen H et al (2020) Concomitant genetic alterations are associated with response to EGFR targeted therapy in patients with lung adenocarcinoma. Transl Lung Cancer Res 9(4):1225–1234
Cheng FJ et al (2021) Cigarette smoke-induced LKB1/AMPK pathway deficiency reduces EGFR TKI sensitivity in NSCLC. Oncogene 40(6):1162–1175
Chetan MR, Gleeson FV (2021) Radiomics in predicting treatment response in non-small-cell lung cancer: current status, challenges and future perspectives. Eur Radiol 31(2):1049–1058
Cruz JA, Wishart DS (2007) Applications of machine learning in cancer prediction and prognosis. Cancer Inform 2:59–77
Cui Y et al (2022) Association of KDR mutation with better clinical outcomes in pan-cancer for immune checkpoint inhibitors. Am J Cancer Res 12(4):1766–1783
Guo Y et al (2020) Concurrent genetic alterations and other biomarkers predict treatment efficacy of EGFR-TKIs in EGFR-mutant non-small cell lung cancer: a review. Front Oncol 10:610923
Hong S et al (2018) Concomitant genetic alterations with response to treatment and epidermal growth factor receptor tyrosine kinase inhibitors in patients with EGFR-mutant advanced non-small cell lung cancer. JAMA Oncol 4(5):739–742
Inoue A (2020) Progress in individualized treatment for EGFR-mutated advanced non-small cell lung cancer. Proc Jpn Acad Ser B Phys Biol Sci 96(7):266–272
Jin Y et al (2018) Mechanisms of primary resistance to EGFR targeted therapy in advanced lung adenocarcinomas. Lung Cancer 124:110–116
Jordan EJ et al (2017) Prospective comprehensive molecular characterization of lung adenocarcinomas for efficient patient matching to approved and emerging therapies. Cancer Discov 7(6):596–609
Kim Y et al (2019) Concurrent genetic alterations predict the progression to target therapy in EGFR-mutated advanced NSCLC. J Thorac Oncol 14(2):193–202
Kourou K et al (2015) Machine learning applications in cancer prognosis and prediction. Comput Struct Biotechnol J 13:8–17
Labbe C et al (2017) Prognostic and predictive effects of TP53 co-mutation in patients with EGFR-mutated non-small cell lung cancer (NSCLC). Lung Cancer 111:23–29
Li X et al (2021) Multi-institutional development and external validation of machine learning-based models to predict relapse risk of pancreatic ductal adenocarcinoma after radical resection. J Transl Med 19(1):281
Liang X et al (2022) Clinical implications of ctDNA for EGFR-TKIs as first-line treatment in NSCLC. J Cancer Res Clin Oncol 149:1211–1220
Lin Y, Wang X, Jin H (2014) EGFR-TKI resistance in NSCLC patients: mechanisms and strategies. Am J Cancer Res 4(5):411–435
Loshchilov I, Hutter F (2018) Fixing weight decay regularization in Adam
Mok TS et al (2009) Gefitinib or carboplatin-paclitaxel in pulmonary adenocarcinoma. N Engl J Med 361(10):947–957
Nguyen B et al (2022) Genomic characterization of metastatic patterns from prospective clinical sequencing of 25,000 patients. Cell 185(3):563-575.e11
Niederst MJ et al (2015) RB loss in resistant EGFR mutant lung adenocarcinomas that transform to small-cell lung cancer. Nat Commun 6:6377
Noronha V et al (2020) Gefitinib versus gefitinib plus pemetrexed and carboplatin chemotherapy in EGFR-mutated lung cancer. J Clin Oncol 38(2):124–136
Offin M et al (2019) Tumor mutation burden and efficacy of EGFR-tyrosine kinase inhibitors in patients with EGFR-mutant lung cancers. Clin Cancer Res 25(3):1063–1069
Pan Y et al (2019) Larger tumors are associated with inferior progression-free survival of first-line EGFR-tyrosine kinase inhibitors and a lower abundance of EGFR mutation in patients with advanced non-small cell lung cancer. Thorac Cancer 10(4):686–694
Peng H et al (2019) Prognostic value of deep learning PET/CT-based radiomics: potential role for future individual induction chemotherapy in advanced nasopharyngeal carcinoma. Clin Cancer Res 25(14):4271–4279
Rodriguez-Canales J, Parra-Cuentas E, Wistuba II (2016) Diagnosis and molecular classification of lung cancer. Cancer Treat Res 170:25–46
Saito H et al (2019) Erlotinib plus bevacizumab versus erlotinib alone in patients with EGFR-positive advanced non-squamous non-small-cell lung cancer (NEJ026): interim analysis of an open-label, randomised, multicentre, phase 3 trial. Lancet Oncol 20(5):625–635
Scagliotti GV et al (2008) Phase III study comparing cisplatin plus gemcitabine with cisplatin plus pemetrexed in chemotherapy-naive patients with advanced-stage non-small-cell lung cancer. J Clin Oncol 26(21):3543–3551
Siegel RL et al (2021) Cancer statistics, 2021. CA Cancer J Clin 71(1):7–33
Skoulidis F, Heymach JV (2019) Co-occurring genomic alterations in non-small-cell lung cancer biology and therapy. Nat Rev Cancer 19(9):495–509
Song J et al (2020) Development and validation of a machine learning model to explore tyrosine kinase inhibitor response in patients with stage IV EGFR variant-positive non-small cell lung cancer. JAMA Netw Open 3(12):e2030442
Soria JC et al (2018) Osimertinib in untreated egfr-mutated advanced non-small-cell lung cancer. N Engl J Med 378(2):113–125
Sung H et al (2021) Global cancer statistics 2020: GLOBOCAN estimates of incidence and mortality worldwide for 36 cancers in 185 countries. CA Cancer J Clin 71:209–249
Tan J et al (2021) The predictive values of advanced non-small cell lung cancer patients harboring uncommon EGFR mutations-the mutation patterns, use of different generations of EGFR-TKIs, and concurrent genetic alterations. Front Oncol 11:646577
Funding
None.
Author information
Authors and Affiliations
Contributions
DS and RG designed this study and directed the research group in all aspects. XL and YC drafted the manuscript. JZ, YM and JZ collected the data. RG and YY provided the statistical software and performed the data analysis. JD and JS arranged the Figures and Tables. LL and WM revised the manuscript. All authors read and approved the manuscript.
Corresponding authors
Ethics declarations
Conflict of interest
The authors declare that they have no conflict of interest.
Ethical approval and consent to participate
The study was approved by the Ethics Committee of the First Affiliated Hospital of Nanjing Medical University, affiliated hospital of Nantong University and Jiangyin People’s Hospital.
Informed consent
Yes.
Registry and the registration
N/A.
Animal studies
N/A.
Consent for publication
Not applicable.
Additional information
Publisher's Note
Springer Nature remains neutral with regard to jurisdictional claims in published maps and institutional affiliations.
Supplementary Information
Below is the link to the electronic supplementary material.
Rights and permissions
Springer Nature or its licensor (e.g. a society or other partner) holds exclusive rights to this article under a publishing agreement with the author(s) or other rightsholder(s); author self-archiving of the accepted manuscript version of this article is solely governed by the terms of such publishing agreement and applicable law.
About this article
Cite this article
Liang, X., Guan, R., Zhu, J. et al. A clinical decision support system to predict the efficacy for EGFR-TKIs based on artificial neural network. J Cancer Res Clin Oncol 149, 12265–12274 (2023). https://doi.org/10.1007/s00432-023-05104-3
Received:
Accepted:
Published:
Issue Date:
DOI: https://doi.org/10.1007/s00432-023-05104-3